In an era where cyber threats are becoming increasingly sophisticated, the role of Cyber Threat Intelligence (CTI) teams is more critical than ever. With the advent of artificial intelligence (AI), the landscape of cyber threat management is undergoing a profound transformation. AI technologies are reshaping how CTI teams gather, analyze, and respond to threats, offering both unprecedented opportunities and unique challenges.
Understanding AI’s Role in Cyber Threat Intelligence
AI, at its core, involves creating systems that can learn from data and make decisions with minimal human intervention. In the context of CTI, AI can be a game-changer in several ways. Enhanced Threat Detection and Analysis Traditional threat detection methods often rely on signature-based systems that can miss new or evolving threats. AI-driven solutions, particularly those utilizing machine learning, can analyze vast amounts of data to identify patterns and anomalies that might indicate a cyber threat. For instance, AI algorithms can analyze network traffic, user behavior, and endpoint activities in real time, flagging potential threats with higher accuracy and speed.
Automated Threat Intelligence Collection AI can streamline the collection of threat intelligence by automating the process of gathering data from various sources, including dark web forums, social media, and other online platforms. Natural Language Processing (NLP) algorithms can sift through massive amounts of unstructured data to extract relevant information about emerging threats, vulnerabilities, and attack vectors.
Predictive Analytics and Threat Forecasting AI can enhance CTI capabilities by providing predictive analytics. Machine learning models can analyze historical data to forecast potential threats and attack trends, allowing organizations to proactively strengthen their defenses. For example, predictive models can assess the likelihood of specific attack scenarios and recommend appropriate mitigation strategies.
Contextualizing Threat Data One of the challenges in cyber threat intelligence is the sheer volume of data that needs to be processed. AI can help by contextualizing threat data, correlating it with existing threat intelligence, and providing actionable insights. This means CTI teams can focus on high-priority threats and make more informed decisions based on comprehensive, contextually relevant information.
Leveraging AI: Practical Applications for CTI Teams
Threat Intelligence Platforms (TIPs) Modern TIPs are increasingly incorporating AI to enhance their capabilities. These platforms can aggregate data from multiple sources, apply AI-driven analytics to identify emerging threats, and automate the distribution of threat intelligence to relevant stakeholders.
Incident Response Automation AI can play a critical role in automating incident response processes. By leveraging AI-driven Security Orchestration, Automation, and Response (SOAR) tools, CTI teams can accelerate the response to detected threats, reducing the time it takes to contain and mitigate incidents.
Advanced Behavioral Analysis AI-driven behavioral analysis tools can monitor user and entity behavior to detect deviations from normal patterns. These tools can identify insider threats, compromised accounts, and other suspicious activities that traditional methods might overlook.
Intelligence Enrichment AI can enhance the value of threat intelligence by enriching it with additional context. For example, AI can cross-reference threat indicators with known attack methods, vulnerability databases, and historical incident data to provide a more comprehensive understanding of potential threats.
Challenges and Considerations
While AI offers significant advantages, its integration into CTI comes with challenges:
Data Privacy and Security Handling large volumes of sensitive data requires stringent data privacy and security measures. Organizations must ensure that AI systems are compliant with regulations and that data is protected from unauthorized access.
Bias and Accuracy: AI models are only as good as the data they are trained on. Biases in training data can lead to inaccuracies in threat detection. Continuous monitoring and refinement of AI models are necessary to maintain their effectiveness.
Skill Gaps: The deployment of AI in CTI requires specialized skills and knowledge. Organizations may need to invest in training or hire experts to effectively leverage AI technologies and interpret their outputs.
Looking Ahead
As cyber threats continue to evolve, AI will increasingly become a cornerstone of effective Cyber Threat Intelligence strategies. By harnessing the power of AI, CTI teams can enhance their ability to detect, analyze, and respond to threats with greater precision and speed. However, it is essential to approach AI integration thoughtfully, addressing potential challenges and ensuring that AI complements rather than replaces the critical role of human expertise in cybersecurity.
In conclusion, the impact of AI on Cyber Threat Intelligence is transformative, offering powerful tools to stay ahead of evolving threats. Embracing AI while carefully managing its challenges will be key to building a resilient and responsive cybersecurity posture in the future.
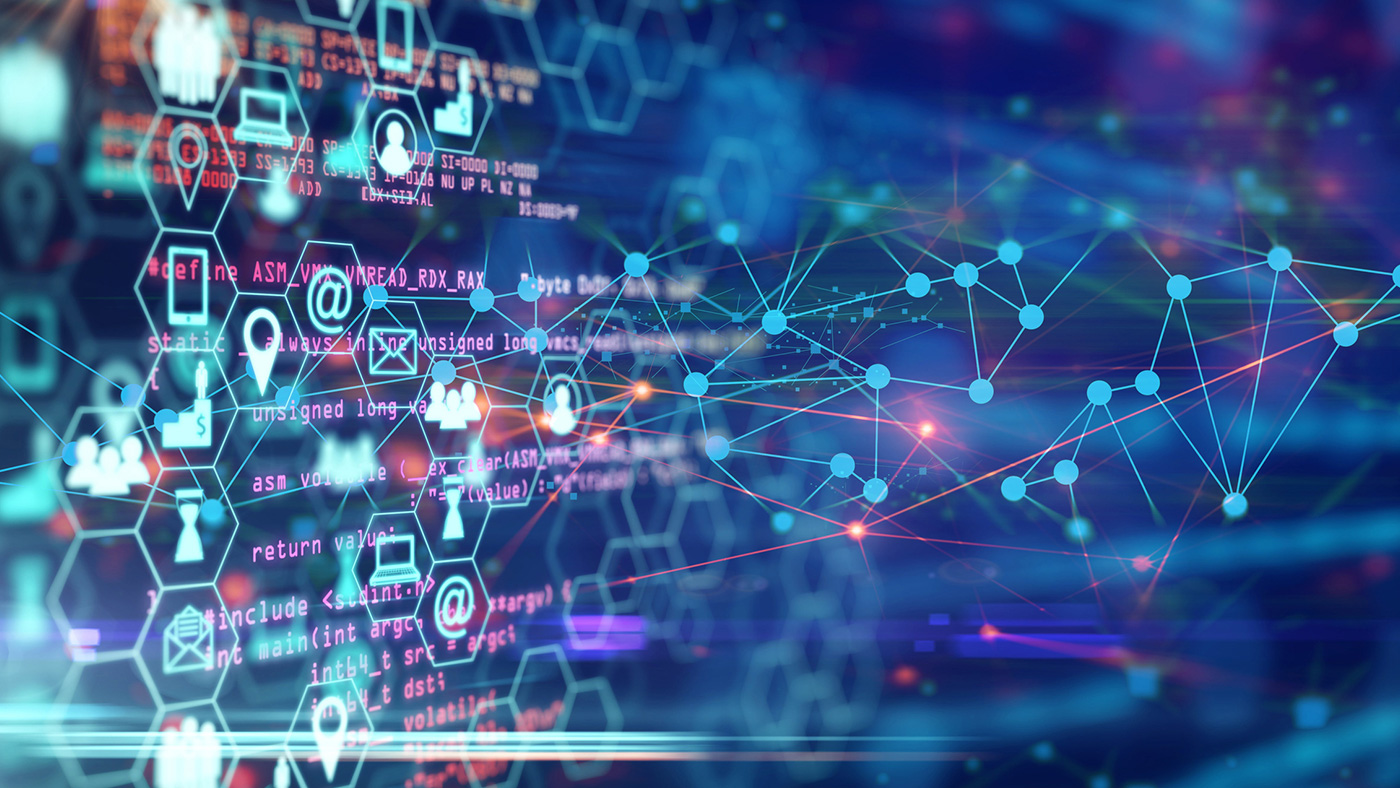